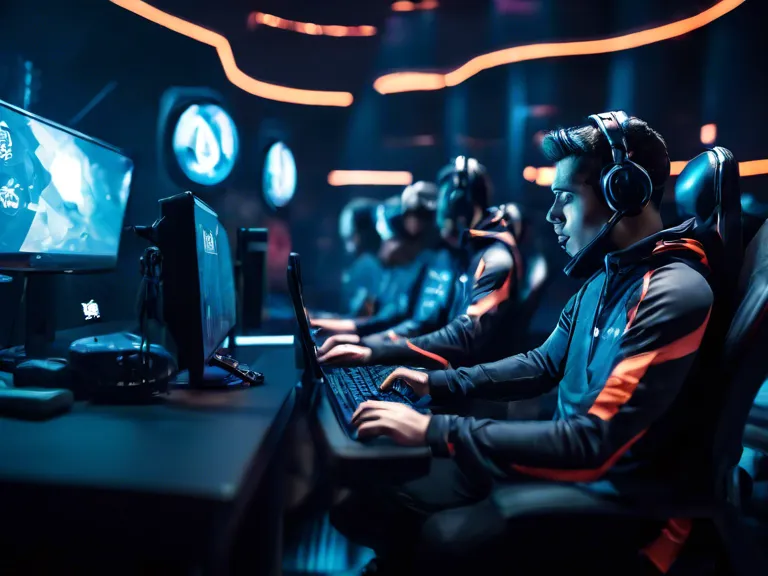
Predictive Data Models in E-Sports: Enhancing Player Performance and Game Strategies
With the rise of data analytics in competitive gaming, e-sports teams are turning to predictive data models to gain a competitive edge. These models utilize a combination of player statistics, game data, and historical trends to forecast outcomes and optimize player performance and strategies.
Predictive data models in e-sports can provide invaluable insights into a player's strengths and weaknesses, helping coaches and analysts tailor training regimens to address areas for improvement. By analyzing data such as kill-death ratios, accuracy percentages, and map control metrics, teams can identify patterns and trends that may not be obvious through traditional methods.
Furthermore, predictive data models can also be used to enhance game strategies. By analyzing opponent tendencies, map preferences, and play styles, teams can develop more effective strategies for both individual matches and tournaments. This predictive approach allows teams to adapt and iterate their strategies based on real-time data, giving them a strategic advantage over their competition.
In addition to player performance and game strategies, predictive data models can also be used to forecast match outcomes and tournament results. By analyzing factors such as team composition, recent performance, and map pool strengths, teams can predict the likelihood of winning specific matches or tournaments. This predictive capability allows teams to make more informed decisions about which opponents to prioritize and how to allocate resources effectively.
In conclusion, predictive data models are revolutionizing the e-sports industry by enhancing player performance, optimizing game strategies, and forecasting match outcomes. As the field of data analytics continues to evolve, e-sports teams that leverage predictive modeling will have a significant advantage over their competitors.